Error signal graph
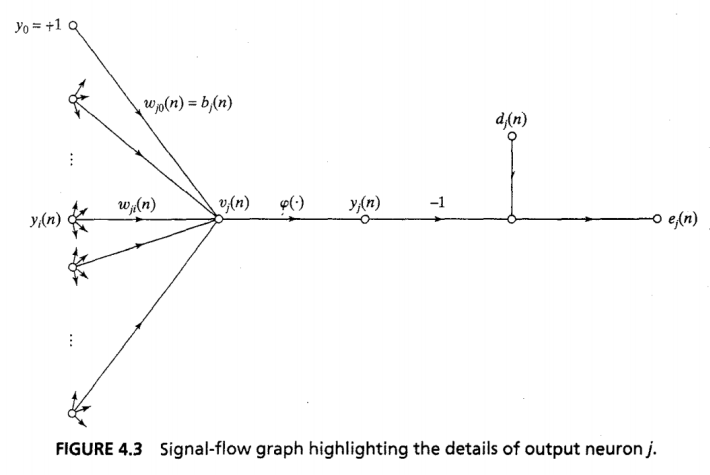
- Error Signal
- ej(n)=dj(n)−yj(n)
- Net Internal Sum
- vj(n)=∑i=0mwji(n)yi(n)
- Output
- yj(n)=φj(vj(n))
- Instantaneous Sum of Squared Errors
- E(n)=21∑j∈Cej2(n)
- C = o/p layer nodes
- Average Squared Error
- Eav=N1∑n=1NE(n)
∂wji(n)∂E(n)=∂ej(n)∂E(n)∂yj(n)∂ej(n)∂vj(n)∂yj(n)∂wji(n)∂vj(n)From 4
∂ej(n)∂E(n)=ej(n)From 1
∂yj(n)∂ej(n)=−1From 3 (note prime)
∂vj(n)∂yj(n)=φj′(vj(n))From 2
∂wji(n)∂vj(n)=yi(n)Composite
∂wji(n)∂E(n)=−ej(n)⋅φj′(vj(n))⋅yi(n)Δwji(n)=−η∂wji(n)∂E(n)Δwji(n)=ηδj(n)yi(n)Gradients
Output Local
δj(n)=−∂vj(n)∂E(n)=−∂ej(n)∂E(n)∂yj(n)∂ej(n)∂vj(n)∂yj(n)=ej(n)⋅φj′(vj(n))Hidden Local
δj(n)=−∂yj(n)∂E(n)∂vj(n)∂yj(n)=−∂yj(n)∂E(n)⋅φj′(vj(n))δj(n)=φj′(vj(n))⋅k∑δk(n)⋅wkj(n)Weight Correction
weight correction = learning rate ⋅ local gradient ⋅ input signal of neuron jΔwji(n)=η⋅δj(n)⋅yi(n)- Looking for partial derivative of error with respect to each weight
- 4 partial derivatives
- Sum of squared errors WRT error in one output node
- Error WRT output y
- Output y WRT Pre-activation function sum
- Pre-activation function sum WRT weight
- Other weights constant, goes to zero
- Leaves just yi
- Collect 3 boxed terms as delta j
- Weight correction can be too slow raw
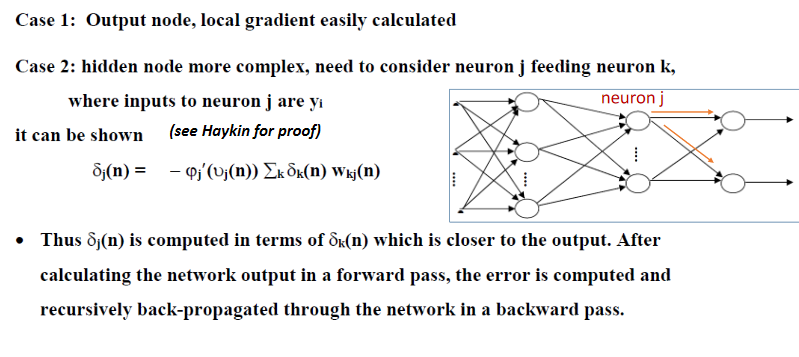
- Nodes further back
- More complicated
- Sum of later local gradients multiplied by backward weight (orange)
- Multiplied by differential of activation function at node
Global Minimum
- Much more complex error surface than least-means-squared
- No guarantees of convergence
- Momentum
- +αΔwji(n−1),0≤∣α∣<1
- Proportional to the change in weights last iteration
- Can shoot past local minima if descending quickly
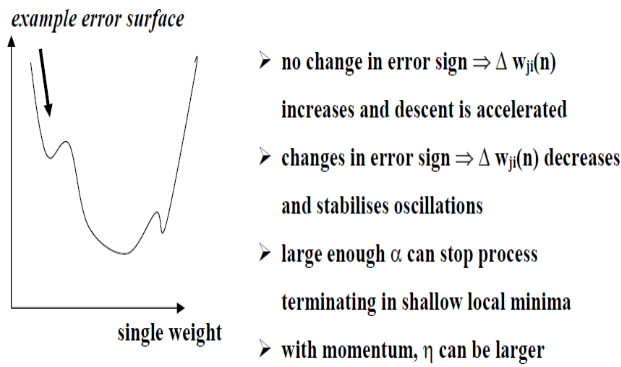
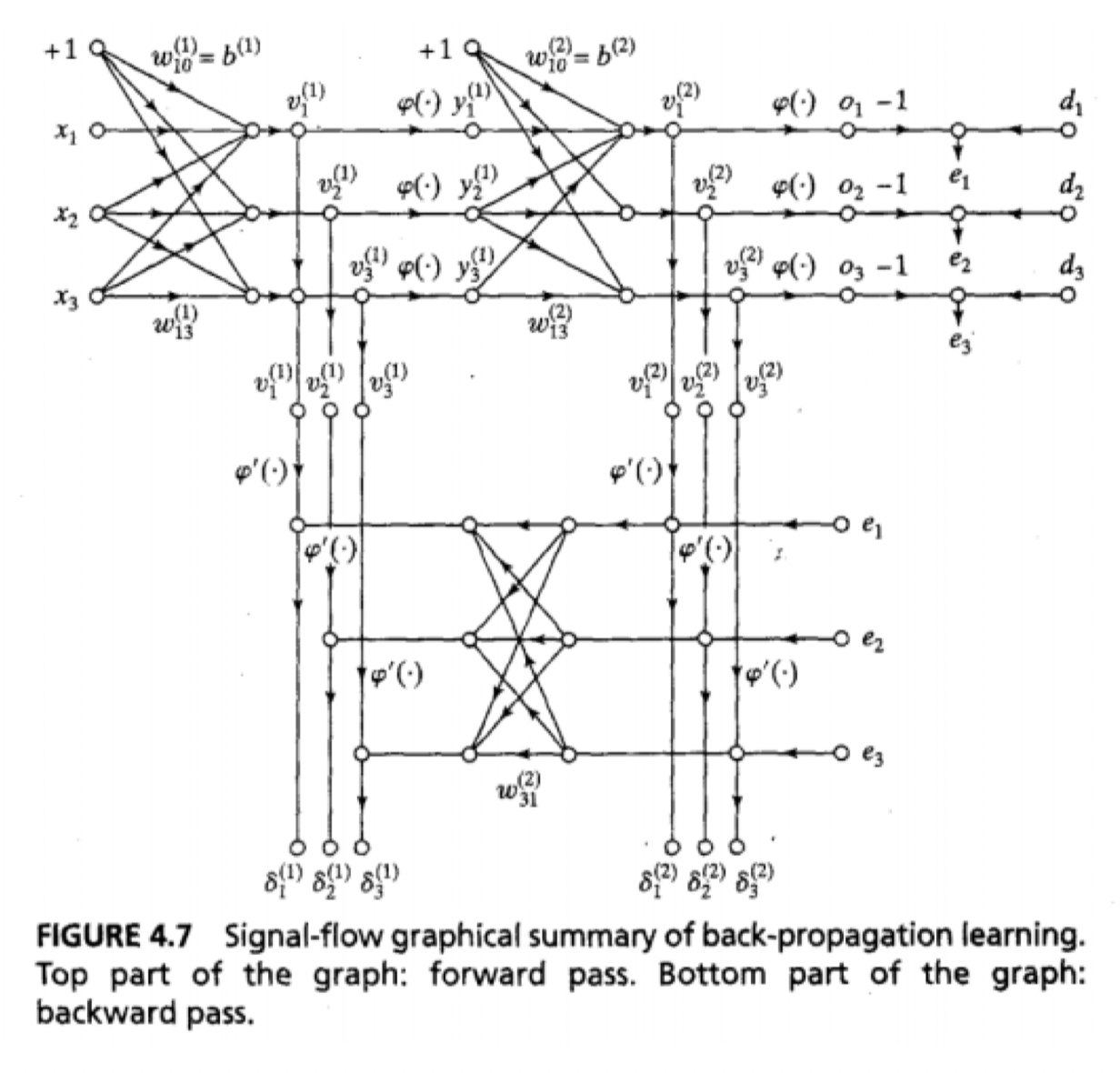
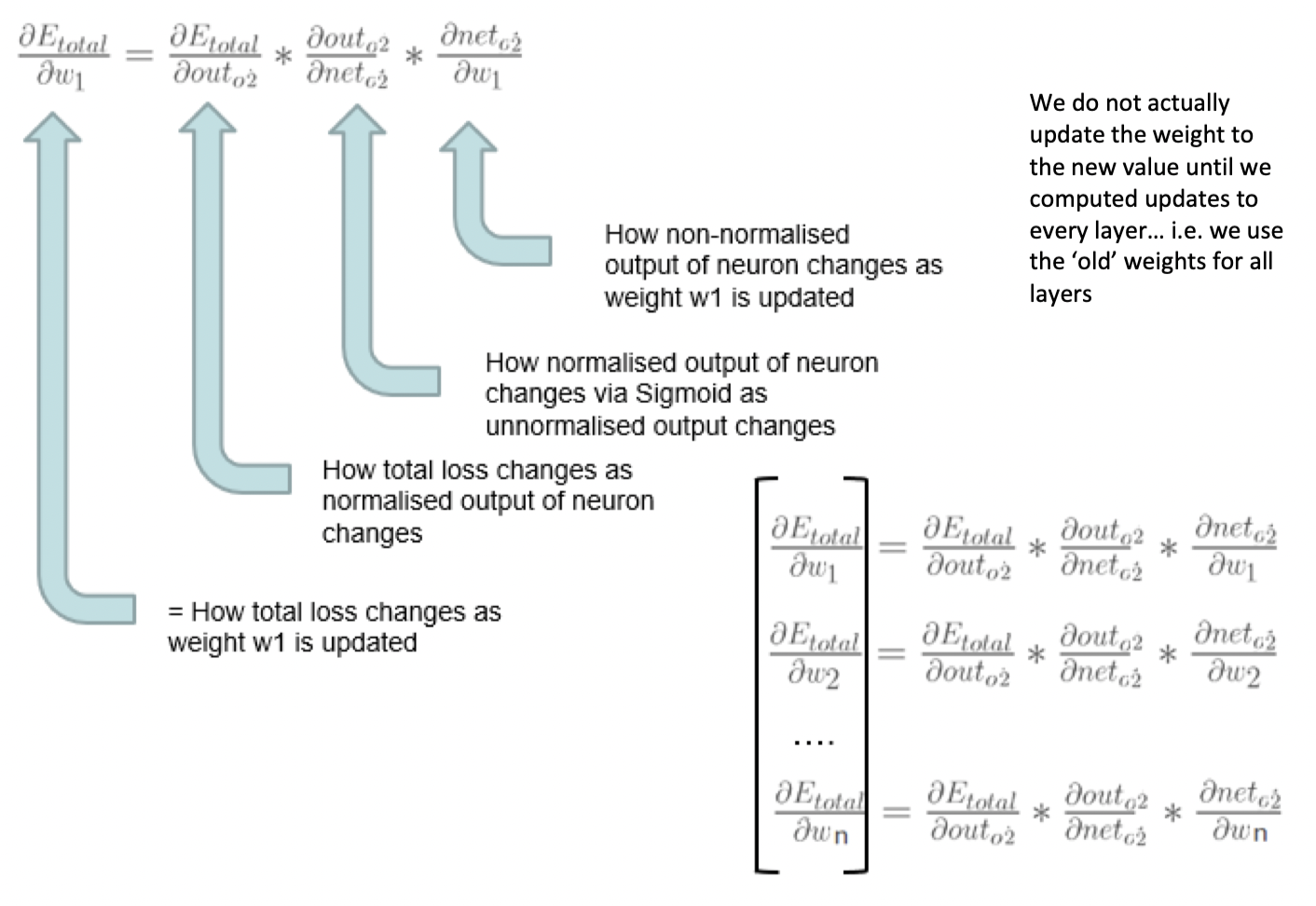
w5+=w5−η⋅∂w5∂Etotal
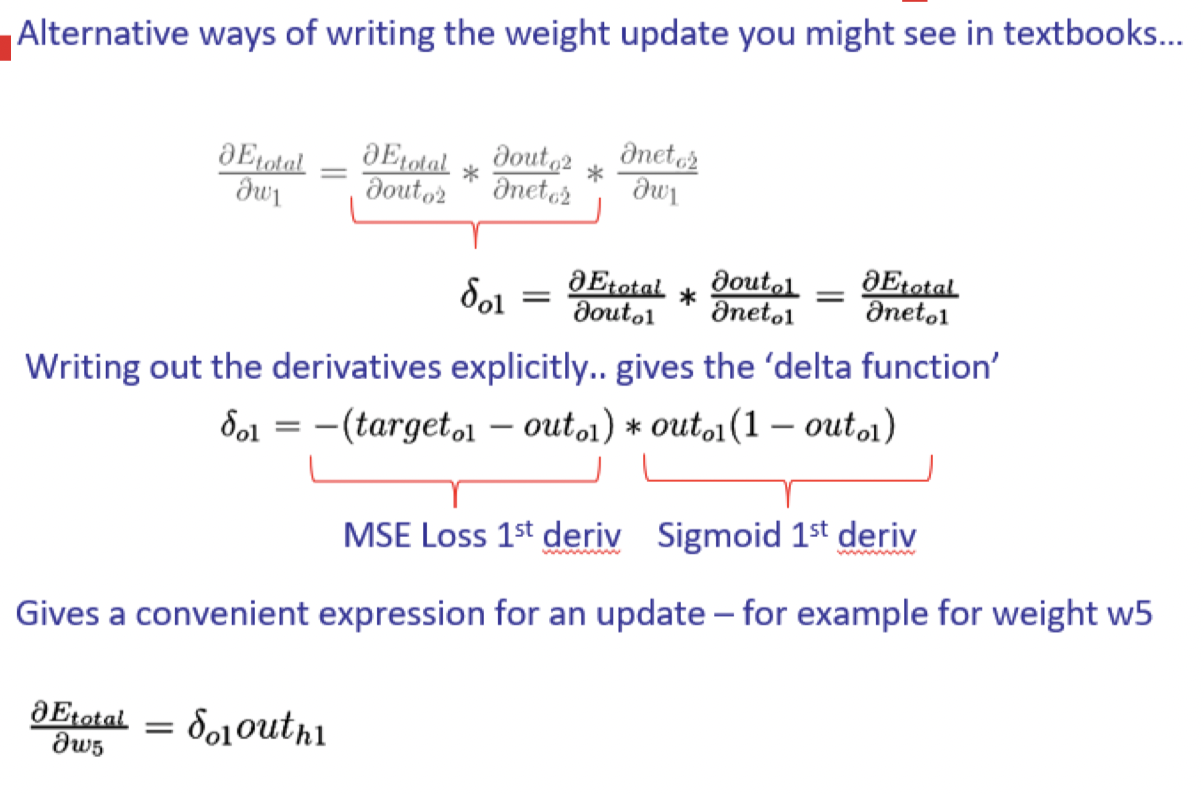