Markov Chains
Hidden Markov Models - JWMI Github
Rabiner - A Tutorial on Hidden Markov Models and Selected Applications in Speech Recognition
- Stochastic sequences of discrete states
- Transitions have probabilities
- Desired output not always produced the same
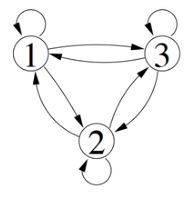
P(X∣M)=(t=1∏Taxt−1xt)ηxTax0x1=πx11st Order
- Depends only on previous state
P(xt=j∣xt−1=i,xt−2=h,...)≈P(xt=j∣xt−1=i)- Described by state-transition probabilities
aij=P(xt=j∣xt−1=i),1≤i,j≤N- α
- For N states
- N by N matrix of state transition probabilities
Weather
A={aij}=0.40.20.10.30.60.10.30.20.8A={πj,aij,ηi}={P(xt=j∣xt−1=i)}Start/End
- Null states
- Entry/exit states
- Don’t generate observations
πj=P(x1=j) 1≤j≤N- Sub j because probability of kicking off into that state
ηi=P(xT=i) 1≤i≤N
- Sub i because probability of finishing from that state
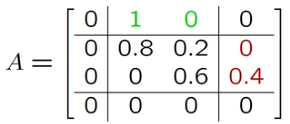
State Duration
- Probability of staying in state decays exponentially
p(X∣x1=i,M)=(aii)τ−1(1−aii)
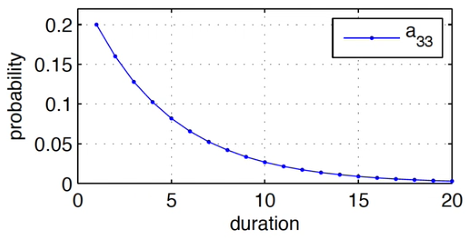
- Given, a33=0.8
- ×0.8 repeatedly